What They Talk About When They Talk About AI
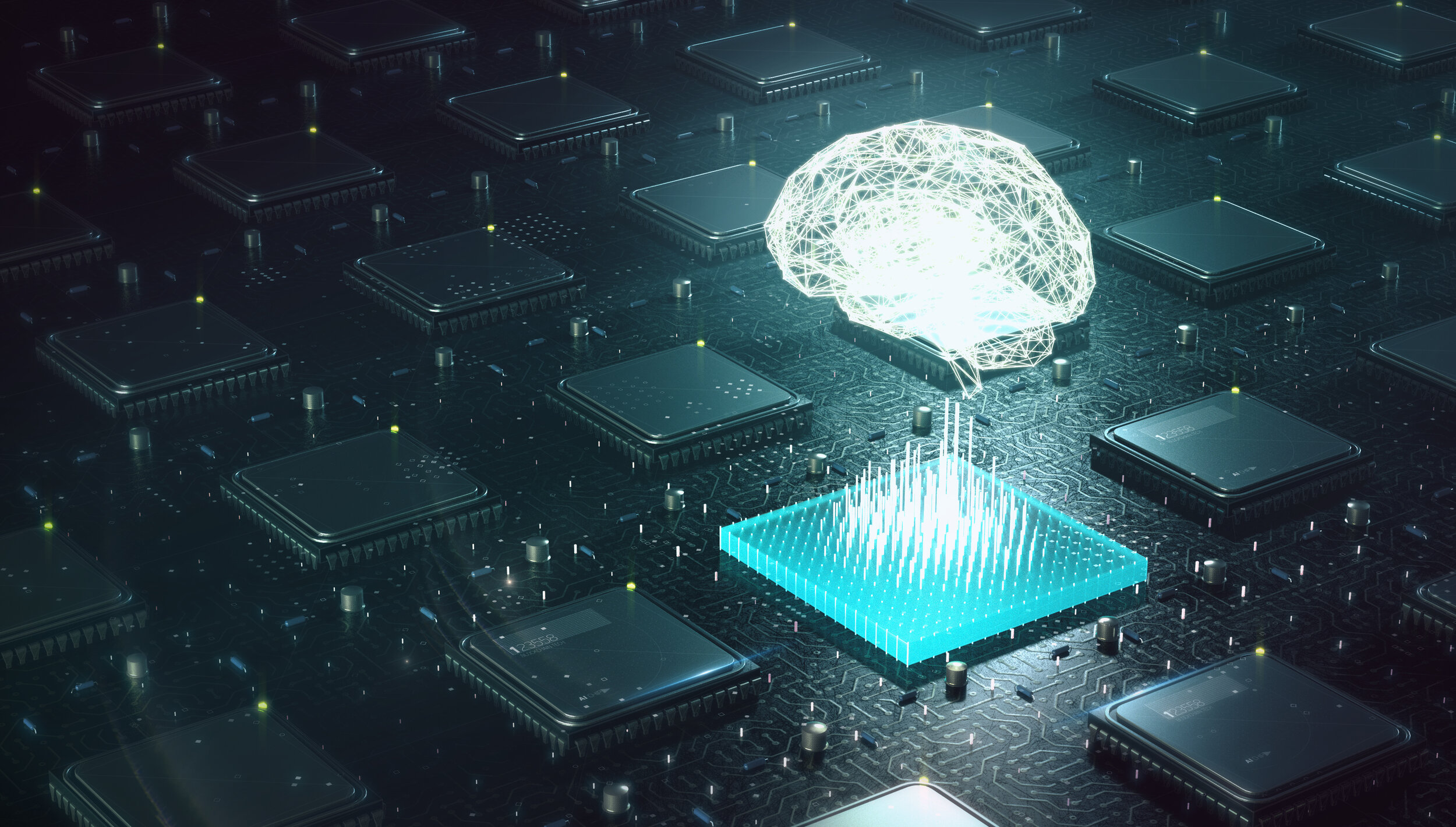
The current data science landscape must appear extremely confusing for companies starting to explore how to better use their data today.
Some applications of Artificial Intelligence have the potential to disrupt entire industries and investors excited by that prospect are channelling capital towards related projects. Obviously, many businesses try attracting a share of that manna and use their powerful marketing voices to do so.
Those marketing messages are probably the main source of confusion. Artificial Intelligence and Machine Learning are complex topics that are not always fully understood by marketers, journalists and other analysts.
The same publications, in their effort to attract money, also instil a sense of urgency. Their message is that it is already late and that something has to be done immediately…
In this post, we try reducing the confusion by coming back to the definitions of some of the main buzz words. We also attempt to calm down those thinking that they are too late by coming back to the history of some of those fields.
Definitions
Let’s start with some high-level definitions from generalist sources.
Artificial Intelligence (AI): “The theory and development of computer systems able to perform tasks normally requiring human intelligence, such as visual perception, speech recognition, decision-making, and translation between languages.” (Oxford Reference)
Machine Learning (ML): “A type of artificial intelligence in which computers use huge amounts of data to learn how to do tasks rather than being programmed to do them.” (Oxford Learner’s Dictionaries)
Deep Learning: “A class of machine learning algorithms that uses multiple layers to progressively extract higher level features from the raw input.” (Wikipedia)
Note the relationships implied by those definitions. Deep Learning is a form of Machine Learning and Machine Learning is a type of Artificial Intelligence. That relationship is clearly illustrated by experts in the field.
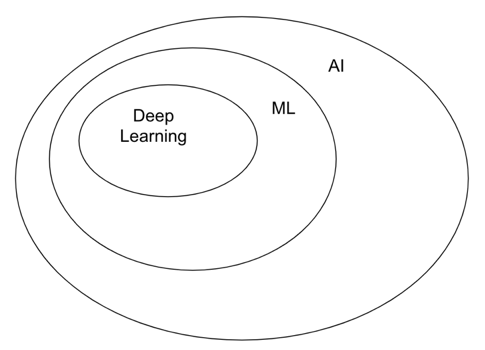
Source: “Deep Learning“, Ian Goodfellow, Yoshua Bengio and Aaron Courville, MIT Press, 2016
It is interesting to note how many articles are opposing some of those techniques and totally missing the point described above. By definition, your ML cannot be more AI than mine! Also, no one is implying any form of hierarchy between different techniques. The tools you will need to tackle your business problems are dependent of the nature of the problem and the available data.
I do not intend to focus on the wider AI in this post. However, I can point you to the rule-based expert systems of the 1980s as an example of what AI without ML looks like. Think about a system that could make diagnostics based on pre-programmed rules related to symptoms.
We can actually start from here to further explain what ML is and how different it is from those traditional programmed systems.
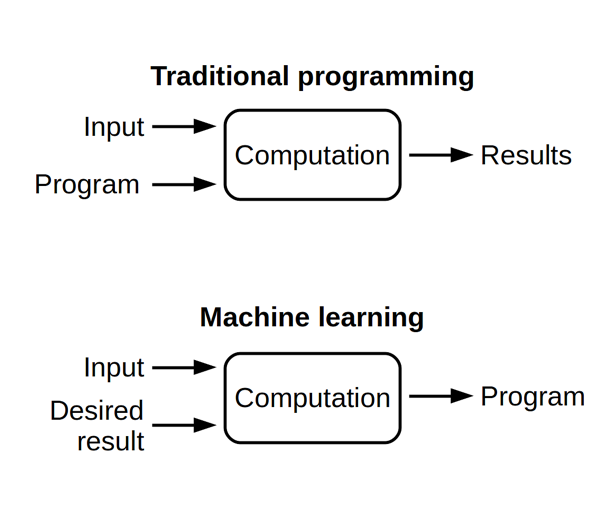
This diagram clearly illustrates what the Machine Learning revolution is all about. Traditionally, programmers had to write programmes fully describing the computations that the machine was supposed to apply to some input data to get the expected results.
Machine Learning is based on the capability of a system to consume input data and data about expected outcomes, to uncover the relationships between the two and to express them as a form of programme that we call a model. No more need for the programmers to think about all the possible rules, the chosen ML algorithm will learn them.
That is a revolution, but one which has started a long time ago!
History
First of all, it is important to remember that much of the Machine Learning we use today is still relying on techniques borrowed from statistics. Think about Ordinary Least Square regression we owe to 18th century mathematicians Gauss and Legendre! But let’s move to a more contemporary vision.
In the 1940s, a combination of new ideas coming from different fields laid the groundwork for the emergence of Artificial Intelligence. Neurology demonstrated that the brain is an electrical network of neurons, cybernetics formalised “the scientific study of control and communication in the animal and the machine” and Alan Turing showed that all computation can be described digitally.
It is finally in 1955 that John McCarty, an American Computer Scientist, coined the term Artificial Intelligence. Arthur Lee Samuel, who developed self-learning Checkers programmes, came with the term Machine Learning in 1959.
The last wave of innovation came in the 2010s when the increase in computing power allowed to fully exploit the Backprogation Algorithm (1986, but based on work started in the 1960s) that describes how to efficiently train Artificial Neural Networks with many layers – that is Deep Learning.
(Note: “Learning Internal Representations by Error Propagation”, Rumelhart, Williams and Hinton (1986))
Deep Learning allowed for the progress in image recognition and Natural Language Processing (NLP) witnessed over the last decade and spectacularly exploited by the major internet companies. And obviously, that wave of successes led to the frenetic marketing activity mentioned earlier.
Where does that leave us?
We are lucky to work in a field that is constantly evolving and where new progresses in Computing and Machine Learning slowly filter into commercial applications. We have a rich palette of tools we can choose from, but we have to keep in mind that the most important question is a business one. What business problem are we trying to address? It is only then that questions about what data to collect or what techniques to use become relevant.