Optimising sales opportunities through Machine Learning
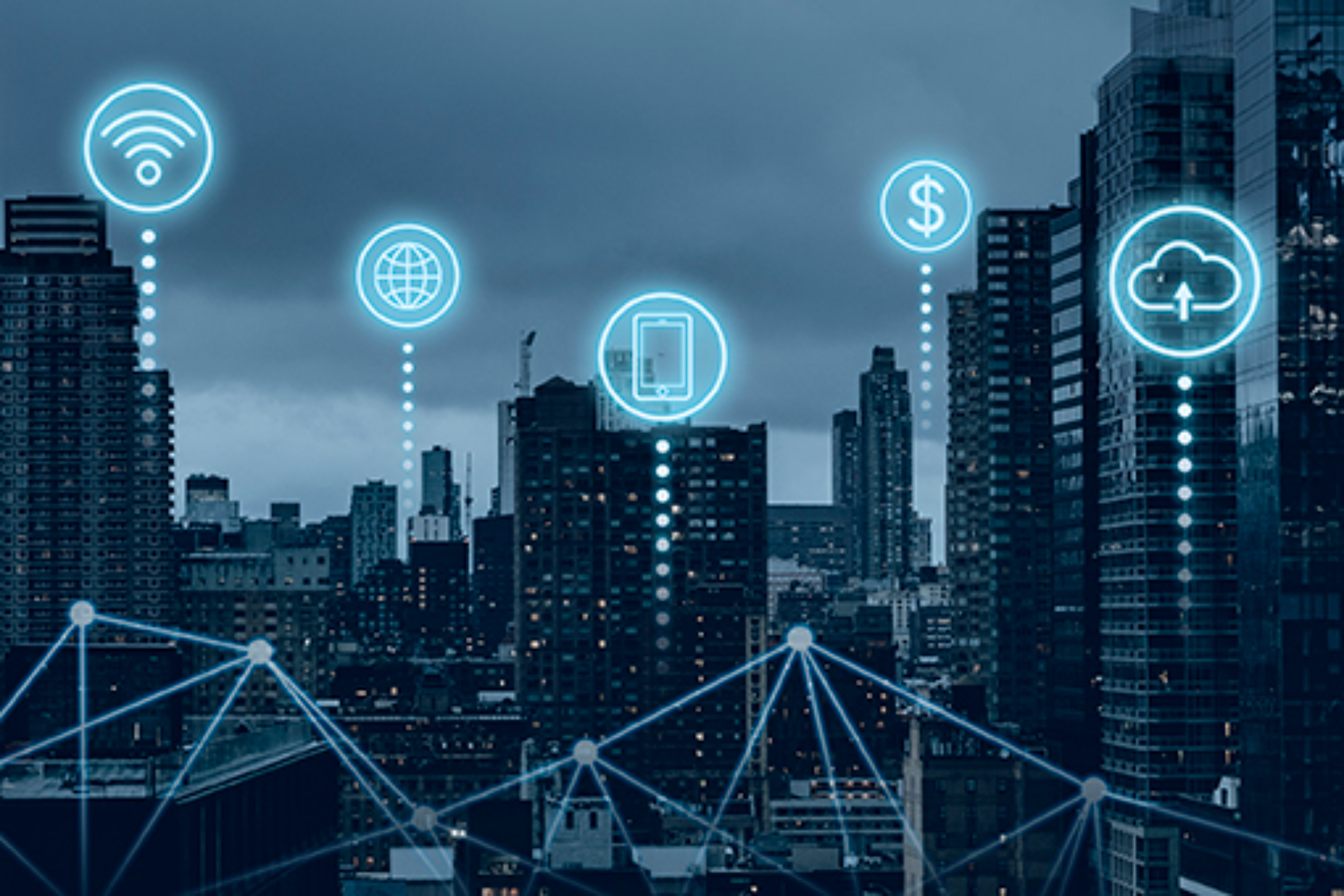
The Project
In order to understand, Station10 consolidated information about the companies (called firmographic data), this intel was provided by Experian and the client’s internal sales figures.
These were used to build a quantile regression model to understand the relationship between a client’s spend and other factors. As the client is the market leader in its main territories, its sales represent an important share of the telco market spend.
The Station10 team built candidate models to predict sales based on features derived from the firmographic data. Then, quantile regression approaches were used to make the model more robust and representative of the entire market.
We then identified the best model by testing a range of “candidate models” on a sample of companies for which the total telco spend is publicly available. Technically, this was done using a quantile random forest regression.
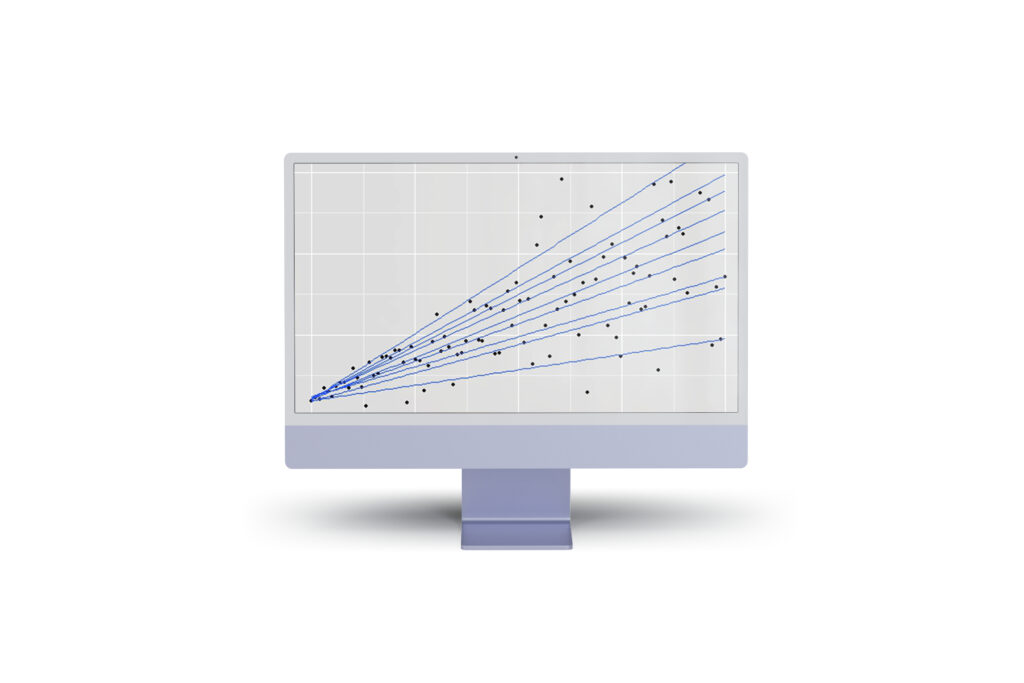
The Challenges
Machine Learning models like this are typically applied to environments where the outcome is already known; there may be many outcomes, but they are still defined upfront. But with this project, the outcomes were unknown, which created a
“learning challenge”, because there would be no feedback loop to tell the model how well it was doing.
This meant the Station10 team were pushing the boundaries of what was possible within the Machine Learning framework. ML isn’t just about creating new ways of doing things, it’s about continuously learning and improving what is already there and extending what has done before into new areas.
One of the other main challenges faced is typical of similar projects where a data-driven model has the potential to replace or sit alongside an existing process based on subjective opinions and intuition. Previously, objectives and targets had been
defined by sales teams and managers themselves, meaning there was a risk of unconscious bias in the data; some sales managers might have suggested easier targets, while others might have set harder ones. This meant there were several data cleansing and classification challenges to ensure such risks were mitigated.
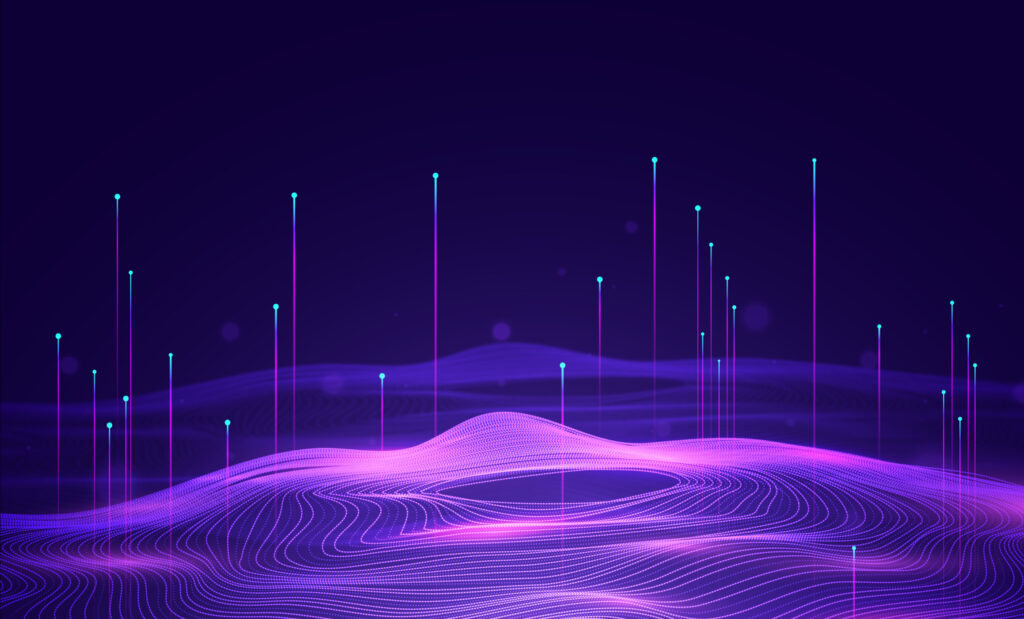
How we overcame them
The first challenge was establishing a data set that was robust and complete enough to provide a full market view. To do this, Station10 used internal data and external firmographic data to model the relationship between company size per industry and the client’s spend using a quartile approach. By validating client spend through external data (Experian) on the details of target companies, the Station10 team could “fill in the gaps” and ensure the data set was robust enough to represent the overall market. Station10 could then fine-tune the model to align with published total market size estimates and used the final model to predict the customers’ wallet size.
However, within that first challenge was also wrapped a second. Because this hadn’t been done before using a data-led approach, the previous sales targets were based on internal perceptions of the market and company spend. Whilst using
external, objective data helped build an accurate view of how to mitigate the risk of the subjective bias from previous target data, the sales teams still needed to understand the information in order to action it and to change their behaviour, if necessary. Therefore, Station10’s data scientists also created a combined dataset to deliver a reporting model, which broke down different sized companies, and different types of classifications, to understand the nuances of the impact of company size. Station10 also worked very closely with a range of teams and stakeholders to ensure the new approach was understood, to assist the change programme teams. Station10 also created data visualisations to ensure the teams could understand the new targets and how they would be shown.
Business Benefits
+ Our work allowed the sales team to organise itself so that it can optimally focus on, and identify, the markets and sectors with the higher potential for growth.
+ It has enabled the enterprises to accurately, and objectively, estimate the potential budget that their corporate customers have to spend on telecoms.
+ It also represents the first time the sales organisation has been able to make data-driven decisions for sales planning and targeting, and so is a key part of their data-led transformation programme across the business.
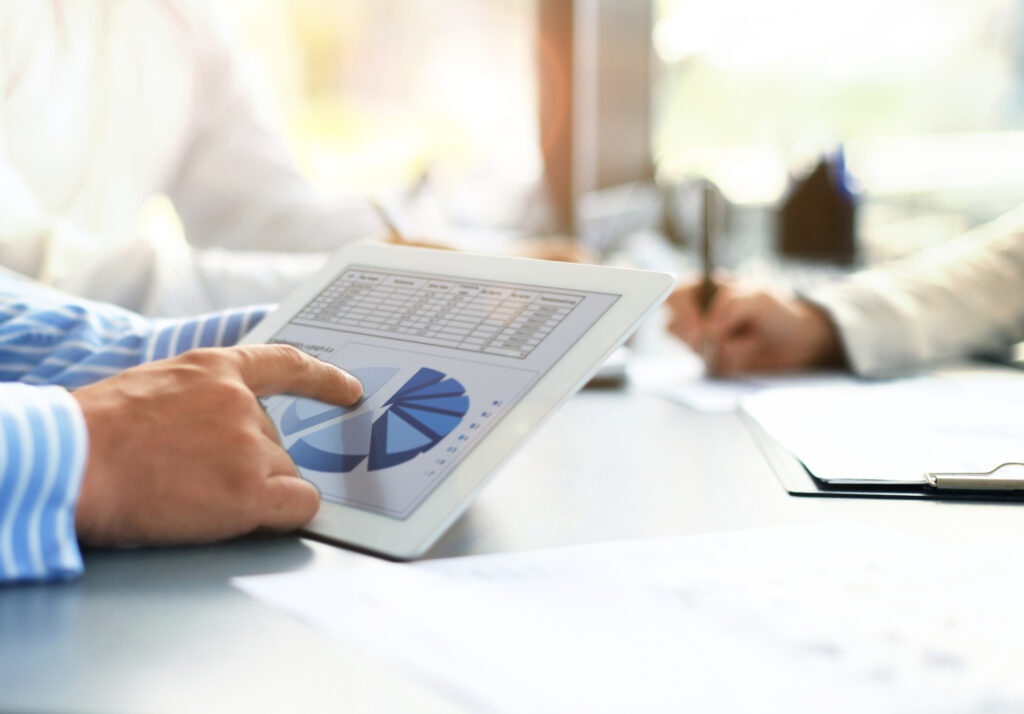