Why focus on data quality in a recession?
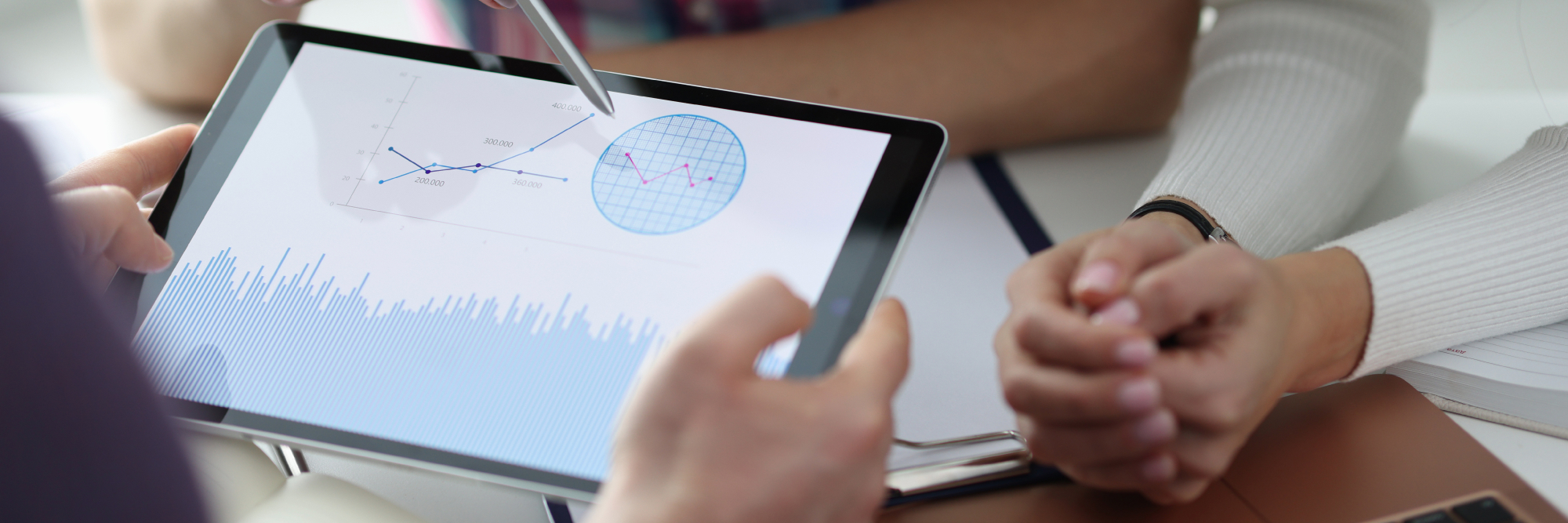
The current economic downturn has magnified the importance of data quality. In tough times, businesses need to be able to rely on their data to make decisions about where to cut costs and how to allocate resources. Poor data can lead to bad decisions that can further hurt a company’s bottom line.
In addition, many businesses are looking for ways to improve their customer relationships. Clean data can help you better understand your customers and what they need from your business. By delivering a better customer experience, you can build loyalty and retention, even in tough economic times.
What are the benefits of clean data?
There are many benefits of clean data, including:
1. Improved decision making
2. Better customer relationships
3. Increased efficiency
4. Reduced costs
5. Improved margins
6. Accelerated growth
Poor data reduces your margins and limits your ability to scale. Data that’s inaccurate or simply not up-to-date prevents you from understanding your customers and delivering the best possible experience. As a result, you miss opportunities, make bad decisions, and end up wasting time and money. When your data is clean, you have a single source of truth that everyone in your organization can rely on.
Clean data also gives you the insights you need to make better decisions, improve your customer relationships, and grow your business.
To get started cleaning up your data, there are a few key things you can do:
1. Conduct a data audit
2. Implement data quality checks
3. Use data cleansing tools and techniques
4. Train your team on data hygiene practices
5. Monitor your data over time
By following these steps, you can begin to clean up your data and improve the quality of information in your organization.
Why is data quality so important to getting value from insight?
There are many reasons why data quality is important to getting value from insight. One reason is that if data is inaccurate, it can lead to false conclusions and decision making. Additionally, data that is not complete can also lead to issues with decision making as key information may be missing. Furthermore, data that is not timely can also prevent organizations from being able to act on insights in a timely manner. Finally, data that is not consistent can lead to confusion and inconsistency in results. Ultimately, all of these factors can result in decreased value from data and insights.
How does poor data quality increase costs?
There are several ways in which poor data quality can increase costs. One way is that if data is inaccurate, organizations may end up making wrong decisions that can lead to wasted resources. Additionally, if data is not complete, organizations may have to expend additional resources to obtain missing information. Furthermore, if data is not timely, organizations may miss opportunities or be unable to take advantage of timely insights. Finally, if data is not consistent, organizations may have to spend significant time and effort trying to clean and standardize the data, which can lead to increased costs.
How does poor data quality decrease revenue?
There are several ways in which poor data quality can decrease revenue. One way is that if data is inaccurate, organizations may make decisions that lead to lost sales or customers. Additionally, if data is not complete, organizations may miss out on opportunities to upsell or cross-sell products and services. Furthermore, if data is not timely, organizations may be unable to take advantage of time-sensitive opportunities. Finally, if data is not consistent, it can lead to confusion and mistrust among customers, which can ultimately lead to lost business.
How can data be inaccurate?
Data can be inaccurate for a variety of reasons. One reason is that it may be measured incorrectly. This can happen if the wrong tools or methods are used to collect data, if data is entered into a system incorrectly, or if there is human error involved in data collection or entry. Additionally, data can become inaccurate over time as things change. For example, a customer’s address may change or a product’s price may fluctuate. If this data is not updated in a system, it can become inaccurate.
How can data be incomplete?
Data can be incomplete if it is not collected properly or if important information is left out. This can happen if data is only collected from certain sources, if data is only collected at certain times, or if data is not collected for all relevant information. Additionally, data can be incomplete if it is not properly processed or organized. This can happen if data is not cleaned up or formatted correctly, if it is not categorized properly, or if it is not linked to other important data sets.
How can data be untimely?
Data can be untimely if it is not collected or processed in a timely manner. This can happen if data is not collected on a regular schedule, if data is not processed quickly enough, or if there are delays in getting data from one system to another. Additionally, data can be untimely if it is not properly managed. This can happen if data is not stored in an accessible or organized manner, if data is not backed up properly, or if there are issues with data security.
How can data be inconsistent?
Data can be inconsistent if it is not collected or processed in a consistent manner. This can happen if data is not collected using the same methods or tools, if data is not processed using the same procedures, or if there are differences in how data is stored or accessed. Additionally, data can be inconsistent if it is not properly linked. This can happen if data is not linked to other important data sets, if there are inconsistencies in how data is linked, or if there are issues with data integrity.
What steps can you take to improve data quality?
There are a number of steps that you can take to improve data quality. One step is to ensure that data is collected correctly. This can be done by using the right tools and methods for data collection, by ensuring that data is entered into systems correctly, and by reducing the potential for human error. Another step is to ensure that data is complete. This can be done by collecting data from all relevant sources, by collecting data on a regular basis, and by ensuring that all relevant information is included. Additionally, you can improve data quality by ensuring that data is timely. This can be done by processing data quickly, by storing data in an accessible manner, and by backing up data regularly. Finally, you can improve data quality by ensuring that data is consistent. This can be done by using the same methods for data collection and processing, by linking data to other important data sets, and by maintaining data integrity.
What is a Data Quality Audit?
A Data Quality Audit is a process of assessing the quality of data. The purpose of a Data Quality Audit is to identify errors, inconsistencies, or other issues with data. Additionally, a Data Quality Audit can provide insights into how data quality can be improved. A Data Quality Audit typically includes a review of data collection, data processing, data storage, and data security. Additionally, a Data Quality Audit may include interviews with key stakeholders, analysis of data quality metrics, and review of data quality documentation.
There are a number of factors that should be considered when conducting a data quality audit. Below is a checklist of some of the most important factors:
– Accuracy: Are the data accurate? Do they accurately reflect what they are supposed to represent?
– Completeness: Are all of the data present? Are there any missing values?
– Consistency: Are the data consistent with each other? Do they follow the same format?
– Timeliness: Are the data up-to-date? Do they reflect the current state of affairs?
– Validity: Do the data make sense? Are they within the expected range?
Web Data Quality Audit Checklist
1. Define the scope of the data to be audited.
2. Identify the source(s) of the data to be audited.
3. Identify the owner(s) of the data to be audited.
4. Identify the intended audience for the data to be audited.
5. Define the criteria for judging the quality of the data to be audited.
6. Collect a sample of the data to be audited.
7. Inspect the collected data for quality issues.
8. Document any quality issues found during the inspection process.
9. Create a plan for addressing any quality issues found during the inspection process.
10. Implement the plan for addressing quality issues.
How often should a Data Quality Audit be conducted?
A Data Quality Audit should be conducted on a regular basis. The frequency of a Data Quality Audit will depend on the size and complexity of the data set, the frequency of data changes, and the importance of data quality. Additionally, the frequency of a Data Quality Audit may be influenced by regulatory requirements or other external factors. In general, it is recommended that a Data Quality Audit be conducted at least annually.
What are some common issues identified in a Data Quality Audit?
Some common issues that are identified in a Data Quality Audit include errors in data collection, inconsistencies in data processing, delays in data availability, and issues with data security. Additionally, a Data Quality Audit may identify opportunities for improvement in data quality management.
What are the benefits of conducting a Data Quality Audit?
The benefits of conducting a Data Quality Audit include improved data quality, reduced costs associated with data errors, improved decision making, and increased customer satisfaction. Additionally, a Data Quality Audit can help to build trust in data and improve organizational transparency.
Free downloadable guide
Our easily digestible guide includes a checklist for your business, as well as answers to FAQs, handy practical tips, and expert insights, including how to ensure your analytical efforts are aligned with your business goals, strategies to attract new customers and improve customer retention rates, and how to use data analytics to cut costs, focus on profit and become more efficient.
We also explore the challenges and opportunities ahead for digital analysts as well as how to integrate online and offline sales data.