The reoccurring theme of data quality
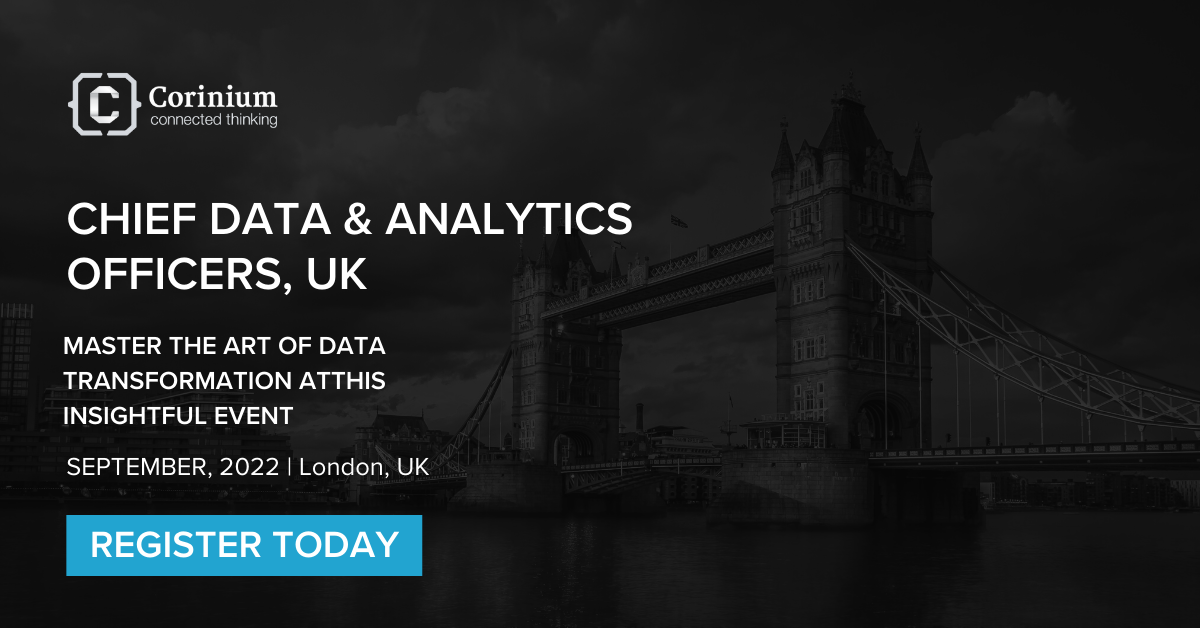
Station10 sponsored the Chief Data and Analytics Officer event in London in September. This was a very interesting conference, in which CDOs, and senior colleagues in data departments, discussed some of their challenges, and opportunities, amongst peers.
There were many takeouts for me, but one that I found particularly noticeable and recurring was Data Quality. As everyone working with data can testify, it’s a very common recurring theme.
I’ve always found the term slightly problematic, for two reasons.
Firstly, data quality is, almost by definition, an outcome of other processes. If you think of a quality assurance process on a production line, the act of ensuring data quality is at the very end of a series of methods of production that created the end product. If there is an issue, you need to identify the nature of the problem, and then fix the relevant method. Data quality is too often discussed as a thing in itself, not an outcome. In reality, it’s the result of a combination of underlying data generation or collection, data management, data ingestion and data engineering.
Put another way, a useful general definition of governance is “the framework of authority and accountability that defines and controls the outputs, outcomes and benefits from projects, programmes and portfolios.” (Association of Project Management). By following this definition, it’s clear that quality is an indicator of the success of the outputs and outcomes of a value-creating process; the way you manage quality is through governance.
It puts me in mind of my cousin, who is a chef. At one point in his career, he was working at the Gramercy Tavern, one of the leading restaurants in New York, as a Quality Assurance Chef. In other words, as an already experienced chef, he was the last person in the kitchen to see and check every plate of food before it went through the door to the restaurant. If he didn’t like the look of it, it didn’t leave the room. Because he understood all of the cooking processes that had gone into creating the plate, he knew whether it was good enough to go out the door. To go to the definition above, he was literally accountable for controlling the output of the kitchen.
My second issue is related to the first; data quality is still a somewhat nebulous term, because it can be subjective. As well as the processes above, it also involves individual’s expectations – phrases like “well, that can’t be right”, or “I don’t believe that”, or even “I don’t like that”, are almost the very definition of subjectivity, and yet they often feature in data quality conversations. To use the analogy of my cousin, the quality assurance process can say “this is good enough to leave the kitchen”, but the diner might not like the dish they ordered. That doesn’t necessarily mean there is a quality issue; it means it wasn’t to the end customer’s taste.
It turns out I’m not alone. Data Quality, and Data Governance, were the two most common underlying topics at the event; if when broader topics came up, there was an underlying conversation about the two related areas. Many people are trying to answer the question – how do you measure data quality? And, to be honest, considering the collective knowledge and understanding, I felt it was harder to answer than it should have been. Several people said they used an external framework by which to be audited every now and again, but then bemoaned the fact that the framework kept changing or being customised, as though the data world, and the Fourth Industrial Revolution (link to Forth industrial revolution blog) that drives it, should have stood still in the intervening period. In addition, one of the metrics discussed for measuring data quality was time to insight. But that’s more of a benefit of the outcome of data quality, rather than a metric of it.
However, I think it’s simpler than that. Data quality is often a phrase that only comes up in a negative context, for something to be fixed, or sent back into the kitchen; not very often does one have a conversation at the end of a project about a “particularly good data quality score”. In the data world, it is possible to quantify this, in a more objective way. A data governance framework should include an indication of the quality of the data sets, including where it came from (provenance), how it was generated, its constituent parts, and when, and when not, it should be used. And a key role of the data governance function is to check the quality of the data before it goes out of the door.
Because, I think the metaphor of the kitchen for a data organisation is an apt one, and it’s one that we often talk about in our Data Practice at Station10. Data-driven insight and data science models are particular dishes on a menu. Like any good chef, it’s important to understand the different ingredients and where they came from. Some ingredients will work for some dishes, and not for others, and some combinations will work well together. The data governance team is a key part of the process – ensuring produce provenance, growing conditions (organic, processed, etc), allergy information, but also that preparation processes are conducted properly, and that it’s checked before it goes out the door.
It’s incredibly important; without it, the restaurant could technically function, but not very well, and certainly not at scale. And in a busy data kitchen, you need a fully planned data governance framework to operate.