Digital Data Quality – The Business Case
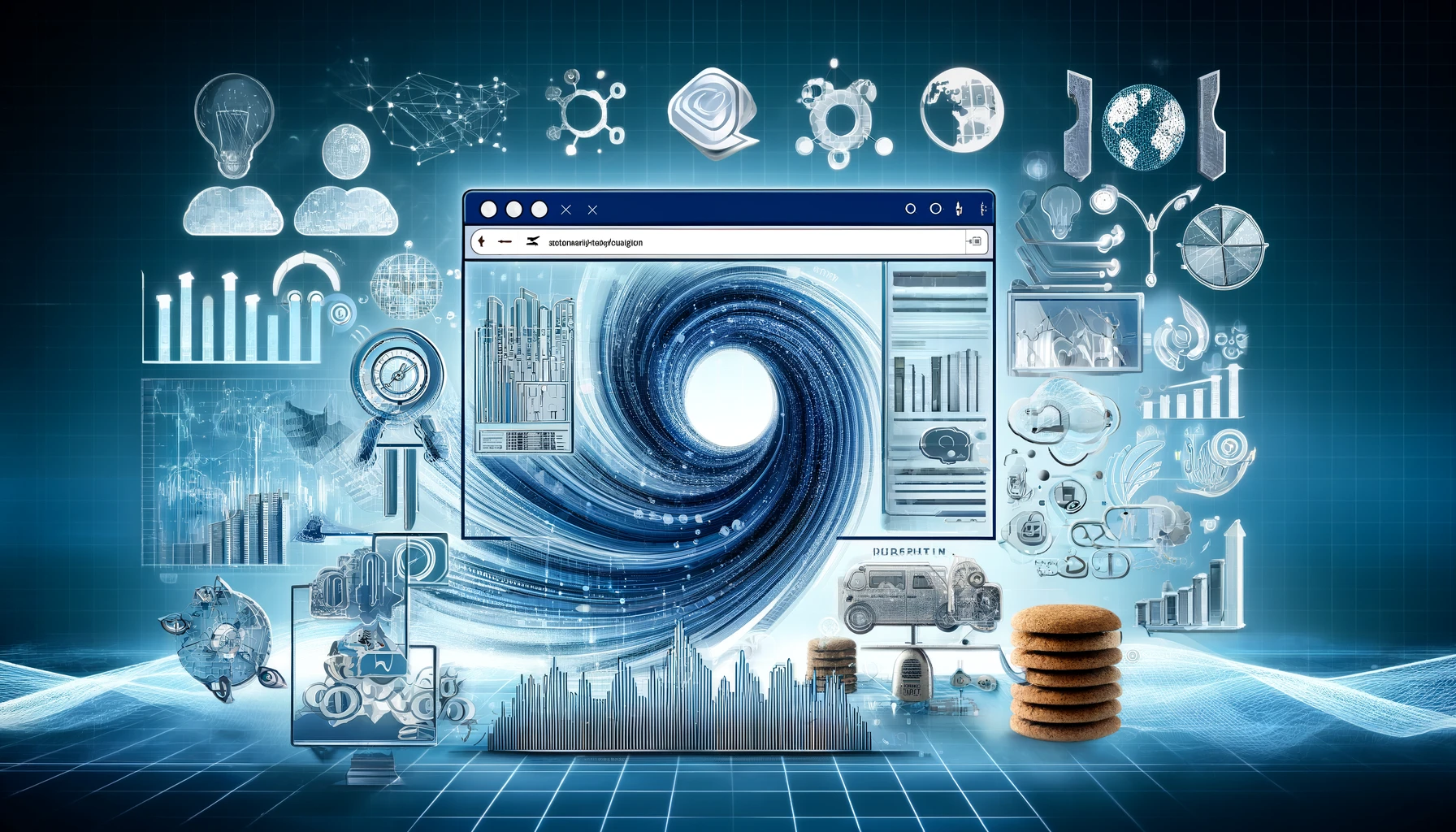
A big focus for Chief Data & Analytics Officers is data quality – ensuring that the data within a business is accurate. This is the very foundation of a successful data organisation; without it, there can be no data trust, and as soon as stakeholders start to lose trust in the data, the CDAO is fighting a losing battle.
For most data sets within the business, structured data and known data boundaries make this task if not straightforward, then at least predictable and can be managed by a more defined process. For instance, if you are looking at transactions, then you will have a known number of them, and the data will follow a pre-defined structured format. There are excellent books on the subject, like Robert Hawker’s one here.
LLMs enable businesses to analyse and manage more unstructured data sets (like text-based environments like web sites or document stores), but again, often the underlying data to be analysed is known, or knowable; at some point, these documents will have been written and gone through a quality review process, or someone can start reading them to check they are relevant and up to standard. Gen AI tools can help with assessing this.
However, digital data quality is often ignored, or assumed to be like structured data sets – defined, knowable, complete. Indeed, it’s often not measured at all by Data Quality or Governance teams. But this misses the point and risks the accuracy of any AI systems built on that data; unlike other data sets, the data set that the business has may well not be the full data set. This can be restricted by privacy limitations by the browser companies, or by consumers themselves. Imagine, for instance, the idea that a consumer could choose to not allow you to record their details in your main finance system of record; it’s not possible, and yet, the equivalent of this is happening all the time in digital data sets.
The real challenge for customer data in an age of AI is data quality. The underlying data has to be good quality for any AI system to work. That becomes even more important with things like Consumer Duty, or the EU AI Act.
Organisations understand that for traditional and structured data sets and data trust. But there seems to be more of a gap with digital. Digital data is structured, but doesn’t behave in the same way as structured data elsewhere in the business.
Last month, at the Adobe Analytics and Appetisers event, Andrew Wathen presented how he has evolved Nationwide’s digital data quality by implementing a new system for Adobe Analytics – AEPSDK. We have also implemented this on several occasions for our clients.
Browsers are increasingly limiting the use of cookies to last for only 7 days. This means that repeat visitors to web sites often show up as new visitors. AEPSDK provides a way to set a first party cookie that can persist for longer than this period, thereby allowing digital data professionals to identify whether an individual is a repeat visitor or not.
This matters, of course, because it’s one of the key building blocks for personalisation of the customer experience. Mainly because it’s only really possible to personalise for a repeat visitor – by definition, you know very little about a new visitor, other than the immediate context of their visit. For personalisation and AI systems to work, you need more information about the customer.
This scenario elegantly demonstrates the unique challenge of digital data quality. Technically, using the old (non-AEPSDK) system is correct and there is nothing you are doing wrong to collect the data – it is collected legally and in line with how the browser operates and allows you to collect data. As a result, from a traditional data quality assessment, it ticks all the boxes; there’s nothing that can be done, so no need for additional time and investment.
But in reality, it doesn’t give the entire picture. Repeat visitors are not correctly detected as such, and so the personalisation and AI efforts – which will have significant investment and focus on them – will not work properly. And so the same data is in fact inaccurate. And therefore the data quality is significantly compromised – whilst being reported as accurate as possible.
That’s why we recommend to all our clients using Adobe Analytics to switch to the AEPSDK version. What was great about Andrew’s presentation, though, was it clearly outlined the impact. Whilst he couldn’t share exact numbers, he could say that this had meant Nationwide were able to identify vastly more repeat customers, who would have otherwise been recorded as new visitors. This meant they were now “personalisable” for the first time, and so this is transformational for the business. (His blog on the topic is due to be published over the summer; I will share it when it is live).
And that’s why digital data quality is so different to any other data set within the rest of the business. There are many more external factors (browsers, consumers, devices, location, sentiment) than can impact whether the data that you collect on your digital customers is truly accurate. That’s why we recommend explicitly and actively managing your digital data quality and accuracy, and also investing in it.
You can start by creating your digital quality metrics. Oh, and if you are using Adobe Analytics, do move to AEPSDK. If you want to discuss what those next steps might be, do get in touch.