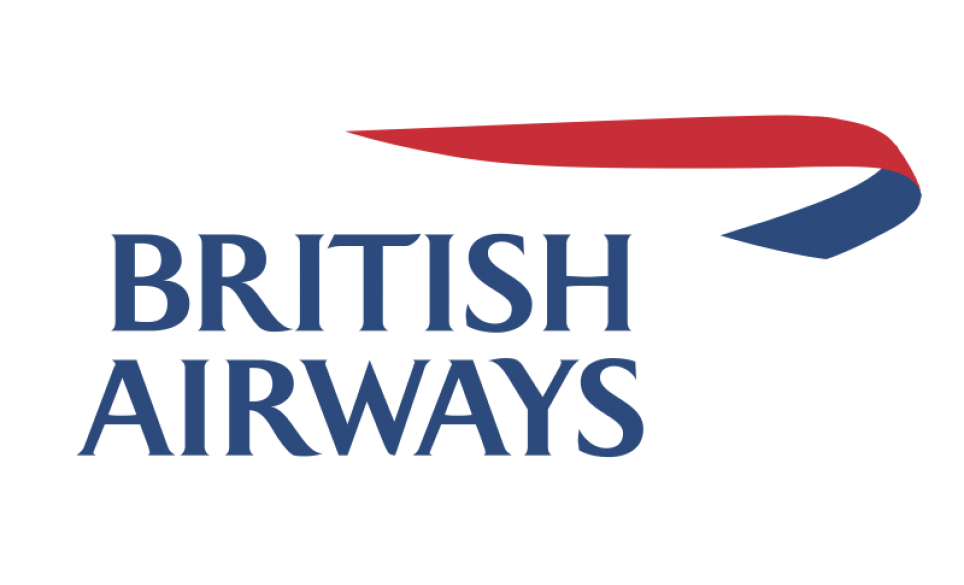
Increasing British Airways’ Cost Efficiencies Through Better Fuel Forecasting
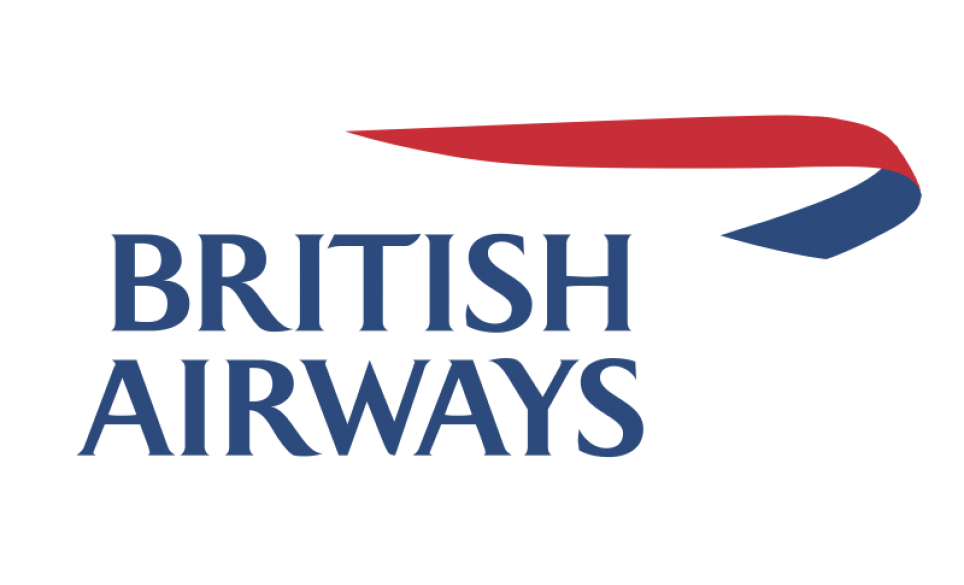
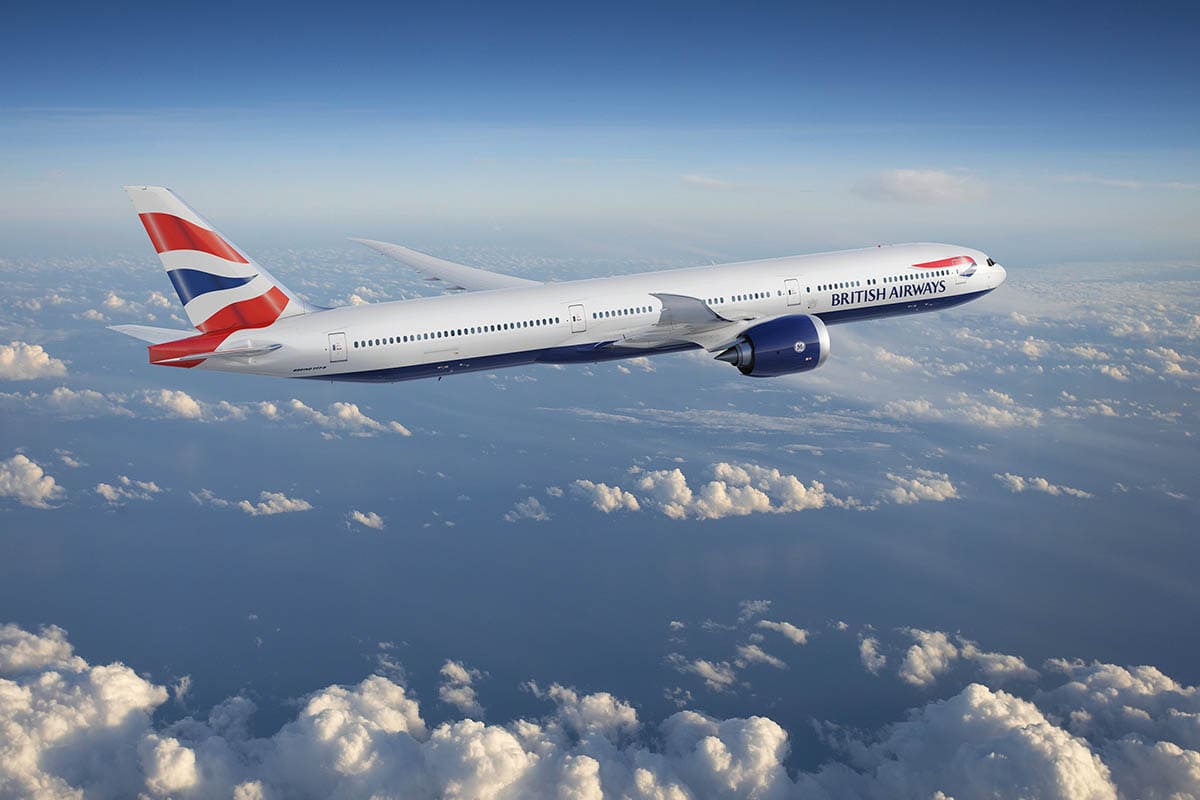
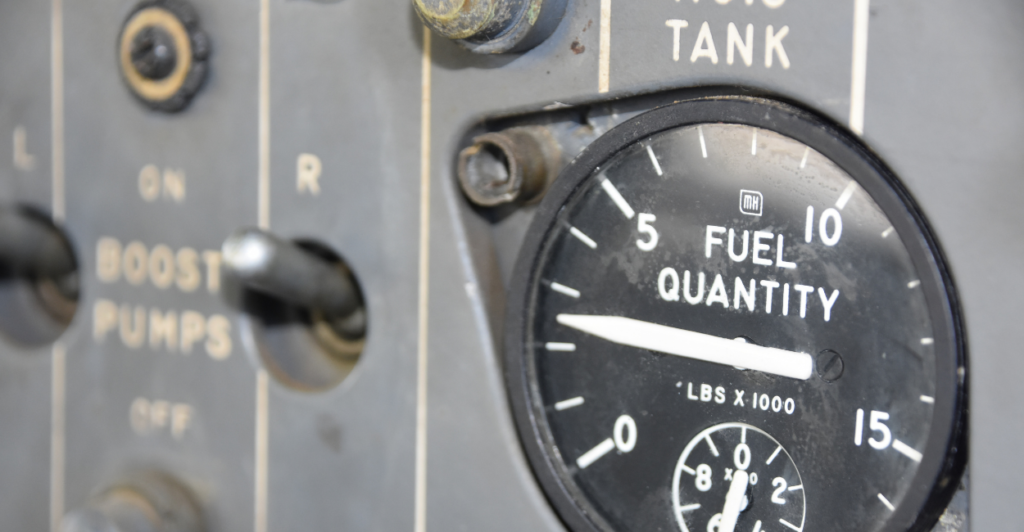
Background
One of an airline’s biggest costs is fuel. Like many others, British Airways buys so much it buys fuel on the futures market to save costs. As a result, it needs to forecast its fuel demands in advance, and does this by calculating the volume of passengers across all routes, globally. Therefore, any improvement on these calculations would have significant cost saving potential for the business.
Historically, these forecasts were taken at a “macro” level, and so were based on total passenger numbers only, meaning passenger booking nuances were not taken into consideration. However, the BA Forecasting team suspected there were additional cyclical factors that influenced these numbers, and so needed to improve the forecast model to encompass these.
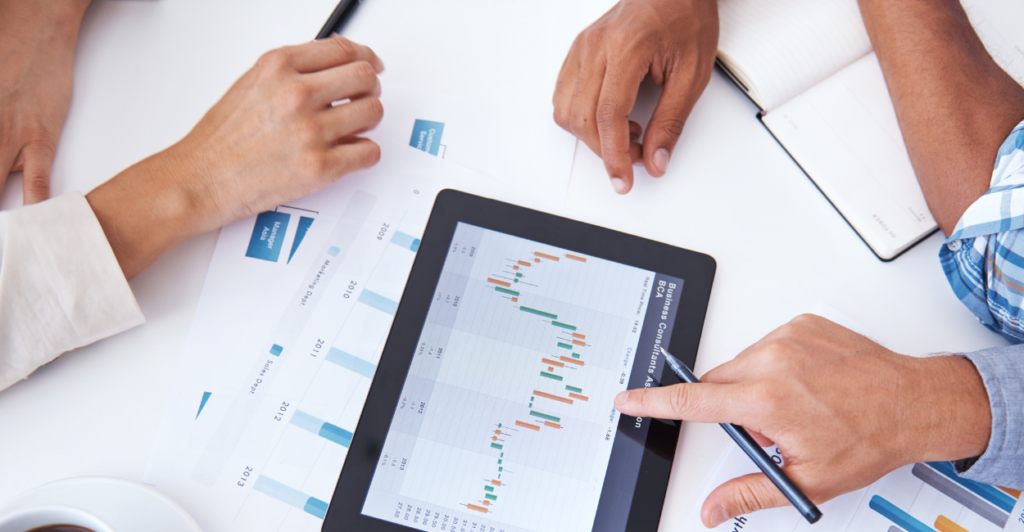
The Project
Station10 was hired by British Airways to investigate how the existing model could be improved, specifically in relation to how holiday periods (Christmas, but particularly Easter) might affect passenger booking behaviour, and how this could be factored into the algorithm to increase accuracy.
Whilst forecasting trends around Christmas may be a comparatively straight-forward task, forecasting the impact of Easter on future fuel markets is a surprisingly difficult task. That’s partly because BA’s forecasting was at a “macro” level, so such nuances had never been analysed before.
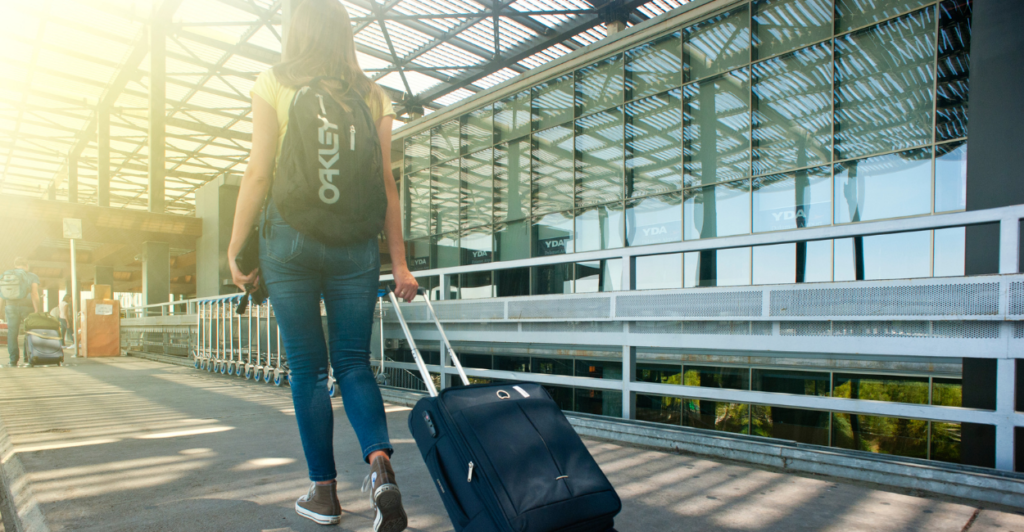
The Challenges
Easter is also a movable feast, so its impact can be very difficult to quantify on passenger trends. Does an Easter in March have a bigger impact on travel than one in April? And how do you “baseline” PAX for those months, when Easter is always going to affect one of them? And does it average out anyway, so the macro figures look the same anyway?
However, the most complex part is the holiday periods themselves. Across the UK (BA’s home market), local school authorities (LEAs) differ their Easter holiday periods, specifically to avoid excessive holiday overcrowding. One school in one village could be on holiday one week, and the one in the next village in a different LEA would be at school.
There was no publicly available single data source for this information, especially for historical data, which is essential for the training data to test the model. This means there is no proxy metric; you just have to gather information for every local authority, over many years, to inform this model.
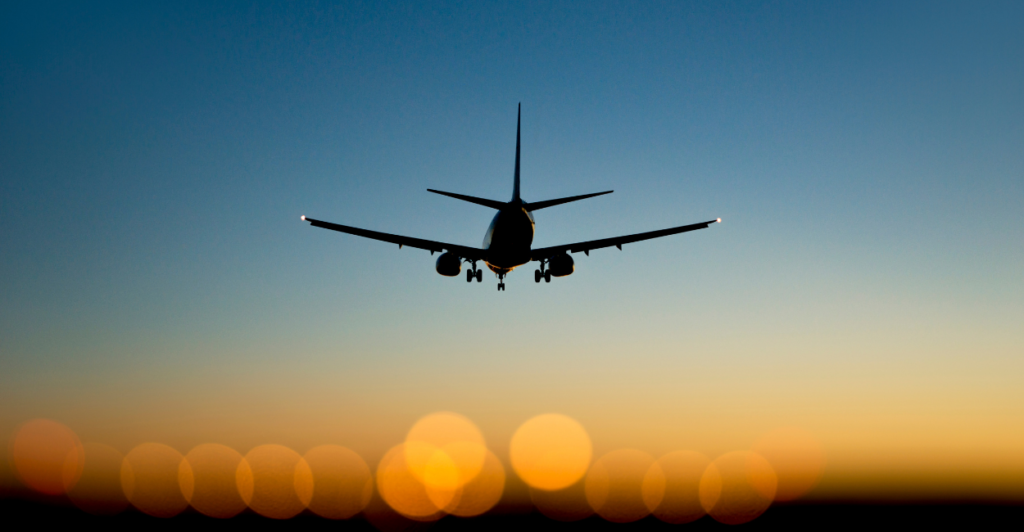
What we did
- Created a school holiday data set for a 5 year period, considering every LEA across the UK
- Created a cohort-based training data set, to build a model and then test this on separate historical data
- Re-analysed the passenger number data “from the bottom up”, rather than top down, to allow us to spot underlying trends in relation to travel types (leisure vs business) and cabin class data.
- Created, ran and tested a series of algorithms to test which combination of variables delivered the most accurate fit
- Discovered some key insights in relation to the :
- Separating out Business and Leisure in the linear model resulted in a significant improvement in the model’s fit and predicted revenue.
- Holiday periods have a significant contributing factor to total revenues, and so including these as factors does increase the accuracy of the model.
- Provided the code to incorporate into the productionised algorithm
The Results
The resulting model delivered a 38% increase in accuracy compared to the previous one. This was worth hundreds of £000’s a year in reduced expenditure on fuel.
Business Benefits
Cost savings of hundreds of £000’s a year from increased algorithm accuracy
Increased confidence and predictability in future costs planning
Additional insights into how booking behaviour can be used to predict future revenues / expenditure