Marketing Attribution: How Multi-Touch Attribution with Markov Chains Can Help Your Business Thrive in Challenging Times
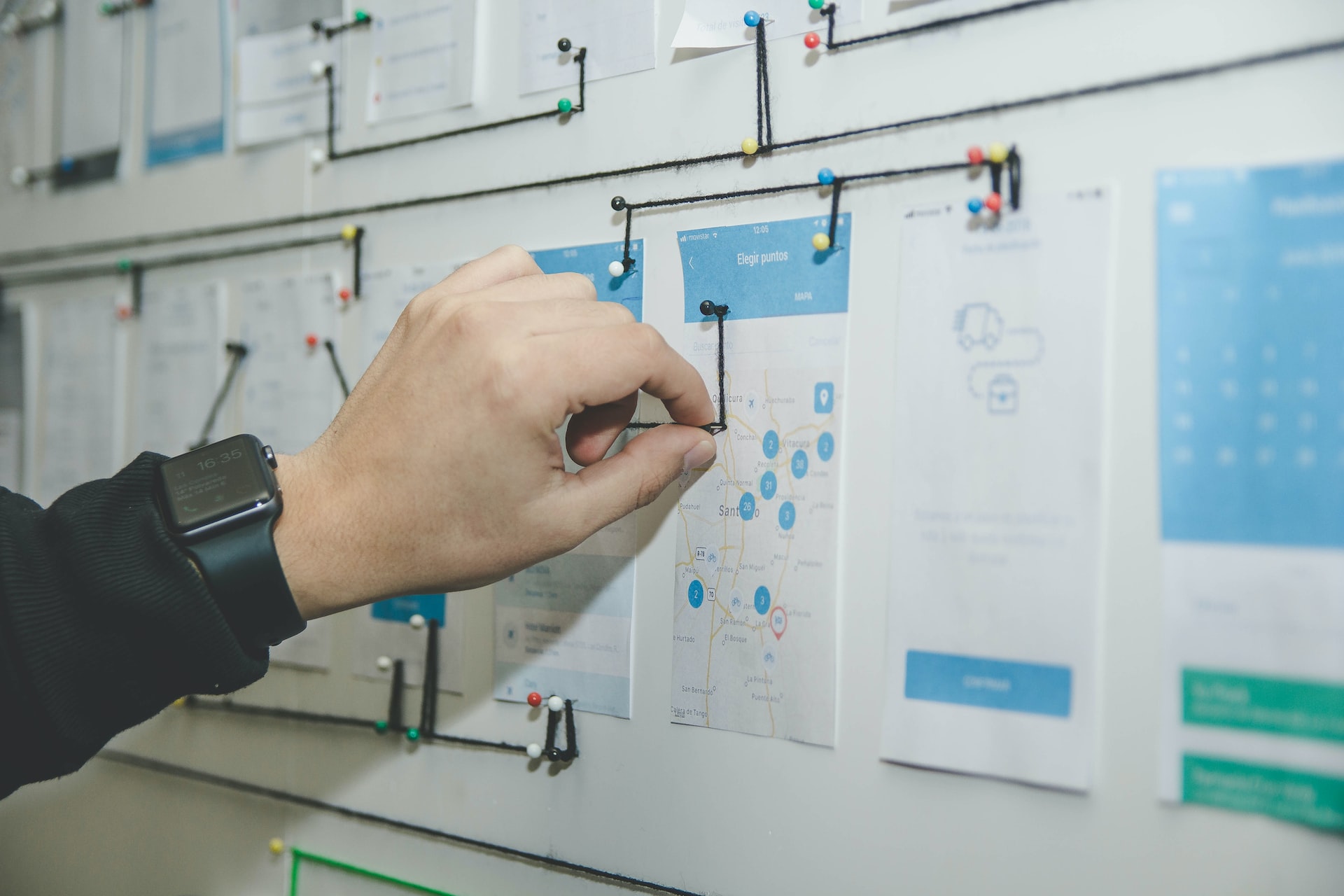
As a marketing manager, you know that in times of economic uncertainty, it is more important than ever to make sure your digital media spend is being used effectively. Use it (effectively) or lose it. Attribution modelling is a vital tool in understanding the impact of your digital media spend, and Multi-Touch Attribution (MTA) is a particularly powerful approach. In this article, we’ll compare MTA with Media-Mix Modelling (MMX), discuss the benefits of both, and explain why MTA can be a game-changer for your business in the current economic climate.
Comparing MTA with MMX
MMX is a well-established methodology that has been used for decades in media planning. It involves a statistical analysis of historical data to determine the relative effectiveness of different media channels in driving business outcomes. However, MMX has some limitations. It can be time-consuming, as it requires a lot of data and can be difficult to interpret, and, by aggregating input data, the nuances of individual journeys are lost.
MTA is an alternative algorithmic approach to attribution modelling. MTA considers the entire customer journey, including all the digital touchpoints that a customer interacts with before making a purchase. Using Markov Chains, MTA can model the complex interdependence between different digital touchpoints, providing a more accurate picture of the relative impact of different media channels. What is the real ROI (return on investment) of Paid Search? Could your Paid Social budget be better spent elsewhere? MTA can start to provide actionable insights for these questions with as little as one weeks worth of data
The mathematical framework for MTA, Markov chains, is not just useful in the context of attribution modelling. It has a wide range of applications across a variety of industries, including finance, physics, and biology. For example, Markov chains can be used to model stock prices, analyse DNA sequences, and rank search engine results.
The Benefits of MTA
MTA has several strengths. Firstly, it provides a more accurate understanding of the impact of different media channels on business outcomes than rule-based models. Rule-based attribution models follow fixed rules for assigning conversion credit. For example, ‘Last click’ gives all credit for the conversion to the last-clicked event. Analytics platforms usually use last click attribution by default. MTA enables businesses to make more informed decisions about where to allocate their digital media spend. Ruled-based models are one-size fits all. Your customers are not.
Secondly, by considering the entire customer journey, MTA allows businesses to identify and optimize those touchpoints that have the greatest impact on customer behaviour. When combined with cost data, these insights allow you to quantify the potential gains from optimisation. When comparing MTA conversion data with last touch, we regularly see the ROI for channels like Paid Search go from positive to negative.
Thirdly, MTA is a robust process. It is not reliant on third-party cookies so is a reliable approach going forward.
Why You Should Start Using MTA today
Fast start: MTA has minimal data setup requirements and does not require a large amount of historical data. This means that you can get your data cleaned up and start modelling fast.
Ideas Factory: MTA can help you uncover actionable insights. No more wasting cash on low-performing channels or ignoring hidden gems.
Reduce Risk: You can make bold changes backed by data-driven insight. When used in conjunction with statistical testing you produce provable outcomes.