How to do cross-device customer analytics – with Peppa Pig!
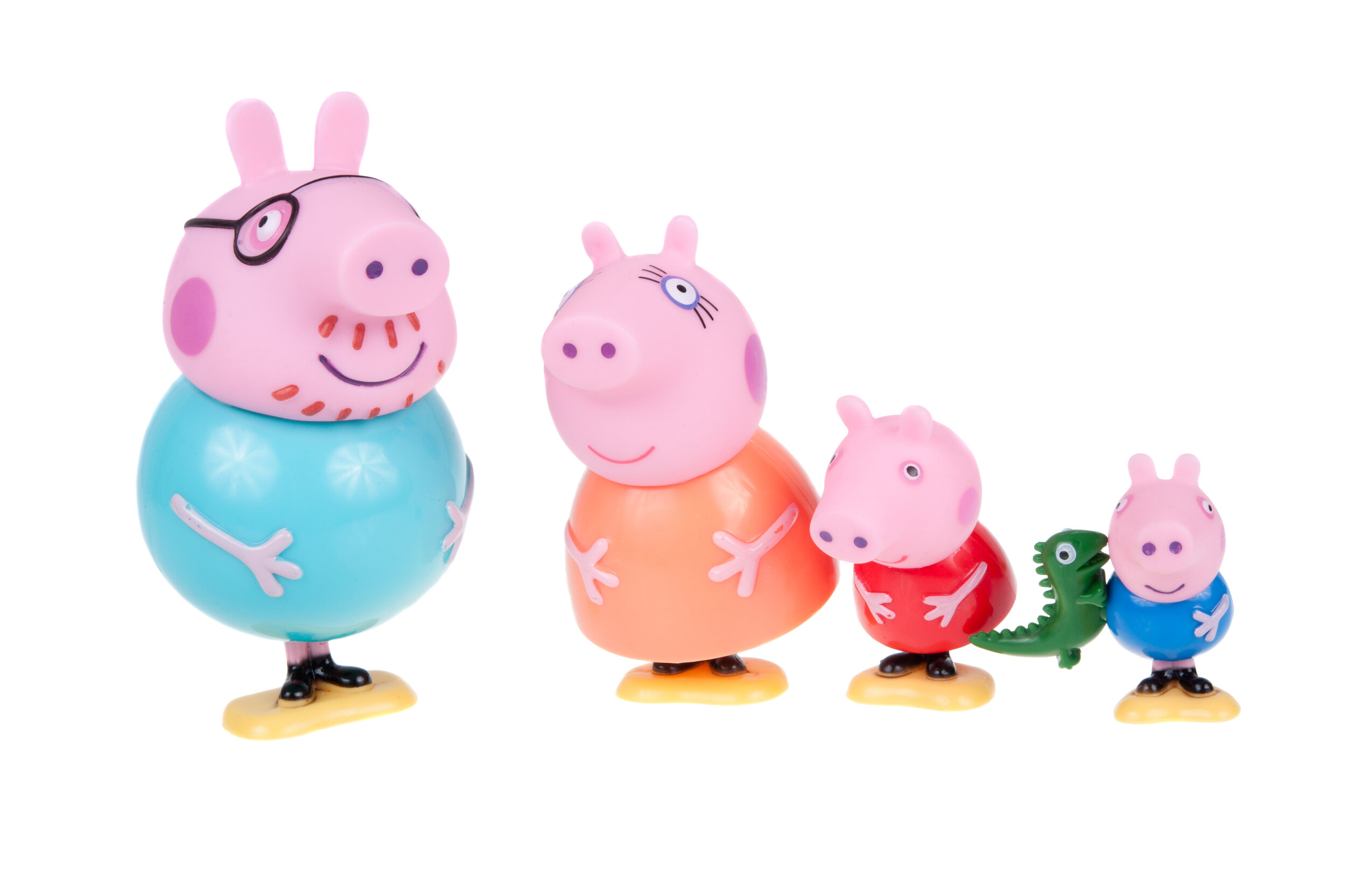
I recall a time when my son was a toddler and we were teaching him a “Join the Dot” Puzzle where you join the dots according to the numerical order to show a picture that only appears clearly when you have, well, joined the dots. It’s pretty self-explanatory, really – a Join the Dot puzzle. Except, of course, if you have never done one before.
He had never done one before but once he got used to the technique of joining the dots it become second nature.
It’s partly a question of being able to count up in the right order, but also to look around (sometimes underneath where your hand is holding the pen) for where the next dot is before you start drawing the line. It’s not exactly rocket science, I grant you, but it is a new skill for those that haven’t done it before.
Many of us are too familiar with Peppa Pig; a young family of anthropomorphised pigs, featuring Peppa, who is in her first year at school, and her younger “toddler” brother George, and Mummy and Daddy Pig.
In one particular episode, Daddy Pig, an architect by trade, is finishing off a blueprint of a house, and uses tracing paper to complete a section of the blueprint, with Peppa and George helping out.
These two examples highlight two challenges with understanding the true value of understanding multichannel customer behaviour, but also usefully point to a metaphor for how to solve this challenge.
“Joining the dots” across omnichannel – computers, mobile and the plethora of other devices – is a challenge that all of our clients continue to face – indeed, if anything, this has become even more important. However, few have really got to grips with the challenge as yet. It is as though we are collectively just learning how to do this particular type of puzzle, and haven’t got the techniques right yet.
However, it is something that the customer experience teams are particularly disadvantaged by, because very often it leads to a partial view of how consumers actually use the products and features they create. For example, customers might conduct product research or even attempt to purchase on mobile, but then complete at home (and so on their computer on the web site) rather than on their mobile or tablet device. This might be either because of perceived security (“I don’t want to get my credit card details where others might see it”) or connectivity considerations (“I am on the move, and therefore will go in and out of signal”).
In my view, this leads to a general, and industry-wide, underestimation of the true business value of multichannel journeys, but particularly those where the primary transaction is less likely to happen – whether that is via apps or mobile web, or any emerging channel. Similarly, this also often leads to an overestimation of the number of customers across all channels. The difficulty, however, is actually proving this difference, because without the ability to join the dots, you can’t even show part of the picture with any accuracy; Going back to the puzzle – if you have no numbers on it, it just remains a random set of dots on a page. As a result, it is likely that it will be the customer experience teams that will need to drive the adoption of these techniques.
There are ways in which this can be done. They imply a fairly robust data capture process and some in-depth analytical tools and resource, but there may be elements of this that can be taken up and used by all companies, at least as a starting point to understand the true impact of all channels better; in my experience, companies often have better quality data than they think they do.
The complete picture – copying Daddy Pig’s tracing paper.
Tracing paper allows us to see the different layers along the same plane; the complete picture builds up when you layer the paper on top in the correct alignment. This is just like how we want to build up a picture of a customer’s multichannel browsing experience; each channel or device is like its own layer of tracing paper which can tell its own story on its own, but only gives the full, correct meaning when it is aligned with all the other channels connected to it.
Just like Daddy Pig’s technical drawing tracing paper, though, for this alignment to be correct, we need some consistent markers on each sheet so that we know when each sheet is correctly lined up. Without these guidelines, we will not know how each channel should correctly match up.
The same principle therefore applies to cross-device channel alignment. Without a marker that appears in the same place in each sheet of tracing paper, we won’t know when things line up. That’s why we need to ensure that we have at least one consistent value set for the same customer across all channels.
Or at least, that’s what we thought. It turns out it is better if there are two markers. But you still have to do a lot of the drawing and tracing yourself.
Originally (we’re talking years ago) the industry thought we could set a consistent Visitor ID value, every time the customer logs in, across any channel, thereby ensuring that this is set as the same for the same visitor, wherever they are. Just as the technology started to catch up with this, so did the legislation, and GDPR, rightly, limited the ubiquity of this, unless customers provided consent.
The reality now is both simpler, and more effort. It’s simpler because the ability to use technology to manage a consistent customer ID as a “key” has advanced, so many solutions can do this, or can be built to do it, so you can track customers in the individual session, and across different devices. At the same time, you need to make sure that you have the right permissions from your customers, and you need to understand, and explain, how you will use the data when you capture it in the first place.
So, the data capture piece is relatively simple, and the explanation and consent takes some planning, and some thinking, which can be harder, but still eminently achievable. A bit like using tracing paper to design a blueprint.
Where the real effort comes in is with the analysis. The data will need to be extracted and analysed separately to come up with the correct models. This requires more in-depth analysis tools and resources who can understand these data sets. This is partly where the recent explosion in data science applications, platforms and techniques can help. A bit like taking a blueprint, and turning it into a real building. Just like Daddy Pig!